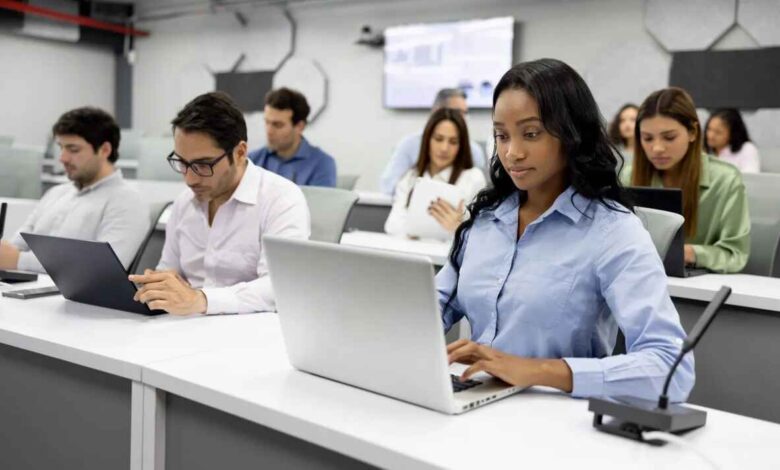
Data Analysis Skills In today’s data-driven world, data analysis skills are indispensable. Businesses, researchers, and professionals across various fields rely heavily on data to make informed decisions, develop strategies, and gain a competitive edge. To excel in this rapidly evolving landscape, mastering data analysis skills is not just beneficial—it’s essential. This article delves deep into the fundamental and advanced techniques that will empower you to harness the full potential of data analysis.
Why Data Analysis Skills Are Crucial in 2024
In 2024, the digital landscape is more complex and data-rich than ever before. Companies are inundated with data from various sources—social media, e-commerce, customer feedback, and more. Data Analysis Skills The ability to analyze this data accurately can lead to significant business insights, driving growth and innovation. Data analysis skills enable professionals to:
- Identify Trends: Detect emerging patterns in the market to stay ahead of the competition.
- Optimize Operations: Streamline processes by understanding inefficiencies and areas of improvement.
- Enhance Customer Experience: Tailor products and services based on data-driven insights to meet customer needs more effectively.
- Drive Decision-Making: Provide a solid foundation for strategic decisions with data-backed evidence.
Foundational Data Analysis Skills
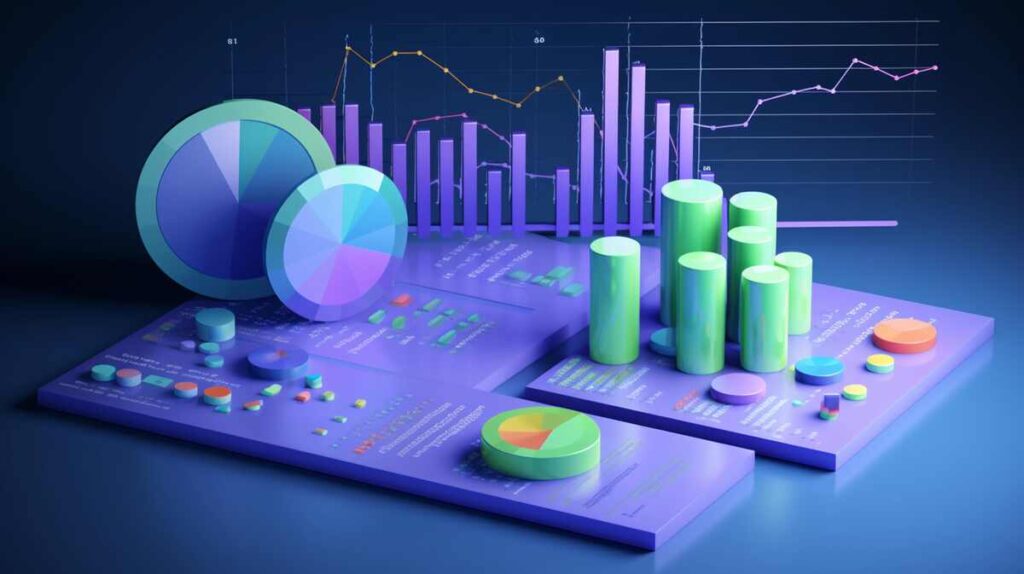
These foundational skills serve as the building blocks for more complex data analysis processes.
1. Understanding Data Types and Structures
One of the first steps in data analysis is understanding the different types of data you’ll encounter. Data can be categorized into:
Examples include sales figures, temperature readings, and time measurements.
- Structured Data: Data that is organized in a predefined manner, such as databases or spreadsheets.
- Unstructured Data: Data that lacks a predefined structure, such as emails, videos, and social media posts.
Understanding these data types is crucial for choosing the right analysis techniques.
2. Data Cleaning and Preprocessing
Before analysis, data must be cleaned and preprocessed. This involves:
- Removing Duplicates: Ensuring that data entries are unique to avoid skewed results.
- Handling Missing Values: Deciding whether to fill in missing data, remove incomplete records, or use other techniques.
- Normalizing Data: Adjusting data from different sources to a common scale without distorting differences in the ranges of values.
A well-prepared dataset is the foundation of accurate and reliable analysis.
3. Statistical Data Analysis Skills
Statistics play a crucial role in data analysis. Key concepts include:
Descriptive Statistics: Summarizing data using measures such as mean, median, mode, and standard deviation.
Inferential Statistics: Drawing conclusions about a population based on a sample of data.
Advanced Data Analysis Techniques
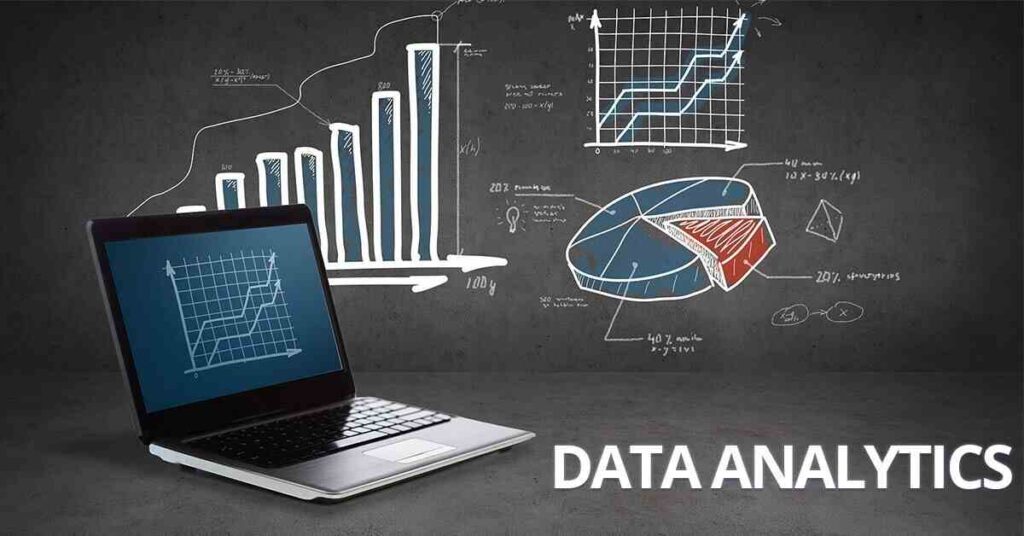
Once you have a solid grasp of the basics, it’s time to explore more advanced techniques that will allow you to uncover deeper insights from your data.
1. Data Visualization
Data visualization is the process of representing data graphically to identify patterns, trends, and outliers. Effective visualization can make complex data more understandable and actionable. Key tools and techniques include:
Bar Charts and Histograms: Used to compare different categories or show the distribution of a dataset.
Heatmaps: Useful for visualizing data in matrix form, often used in market research and consumer behavior analysis.
Dashboard Tools: Platforms like Tableau, Power BI, and Google Data Studio allow for the creation of interactive dashboards that provide real-time insights.
2. Machine Learning and Predictive Data Analysis Skills
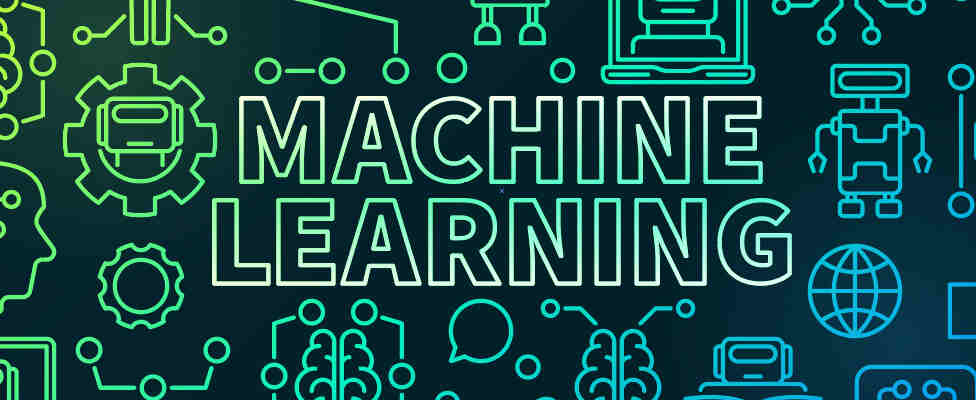
Machine learning (ML) is revolutionizing data analysis by enabling predictive analytics—using historical data to predict future outcomes. Key concepts include:
Unsupervised Learning: Involves training a model on data without predefined labels. Techniques such as clustering and association analysis fall under this category.
Neural Networks: Complex models that mimic the human brain’s neural connections to identify patterns and make predictions.
3. Big Data Analytics
With the exponential growth of data, traditional data processing tools are often inadequate. Big data analytics involves the use of advanced tools and techniques to handle vast amounts of data. Key components include:
- Hadoop and Spark: Frameworks for distributed storage and processing of large datasets.
- NoSQL Databases: Such as MongoDB and Cassandra, designed to handle unstructured and semi-structured data.
- Real-Time Analytics: The ability to process and analyze data as it is generated, enabling instant insights.
The Future of Data Analysis Skills
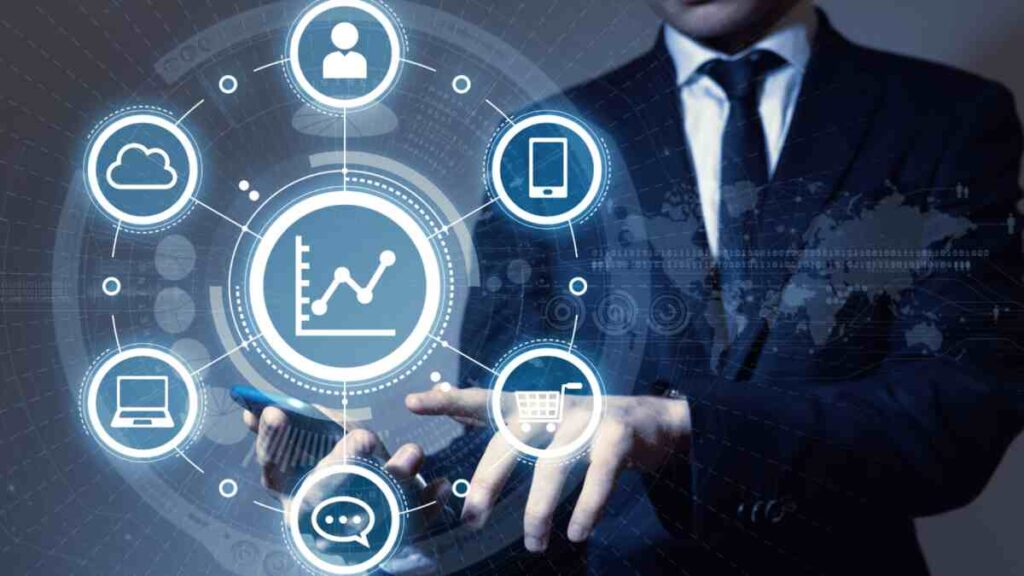
As technology advances, the scope and impact of data analysis will continue to grow. The integration of artificial intelligence, the Internet of Things (IoT), and blockchain technology with data analysis tools will create new opportunities and challenges. Data Analysis Skills Professionals with strong data analysis skills will be at the forefront of innovation, driving progress in every industry.
Conclusion
Mastering data analysis skills is no longer optional—it’s a necessity in the digital age. From understanding basic concepts to leveraging advanced techniques like machine learning and big data analytics, the ability to analyze data effectively is a powerful asset. As we move further into 2024 and beyond, these skills will continue to be in high demand, shaping the future of businesses and industries around the world.
Learn More: Marketing Analytics Tools